Signed in as:
filler@godaddy.com
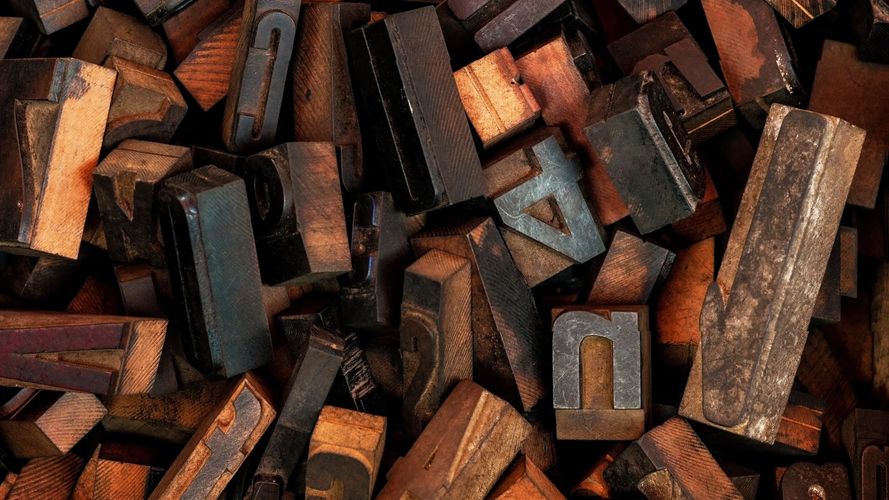
Signed in as:
filler@godaddy.com
I'm Librarian Emeritus at the University of Guelph where for many years I was the Chief Librarian and Chief Information Officer (CIO). Recently I graduated with a PhD from the Faculty of Information and Media Studies, Western University. Researching explainable AI (XAI), algorithmic literacy, and other fun stuff.
Exploring the Information Ecology
Ridley, M. (2024, November 6). Looking backwards to see ahead: The case of expert systems development in libraries. Information Matters, 4 (11). https://dx.doi.org/10.2139/ssrn.5024825
ABSTRACT
This short piece recommends looking at expert system development in libraries during the 1980s & 1990s as a way to advance current AI/ML developments. It is based on the paper in Library & Information History 40.1 (2024): 46–67
**
Ridley, Michael (2024). Informing Algorithmic Literacy Through User Folk Theories. College and Research Libraries, 85(7), 1-12.
ABSTRACT
As part of a broader information literacy agenda, academic libraries are interested in advancing algorithmic literacy. Folk theories of algorithmic decision-making systems, such as recommender systems, can provide insights into designing and delivering enhanced algorithmic literacy initiatives. Users of the Spotify music recommendation systems were surveyed and interviewed to elicit their folk theories about how music recommendations are made. Seven folk theories emerged from this study and are grouped into four themes: agency, context, trust, and feelings. These four themes are used to illustrate how folk theories can inform algorithmic literacy programming and curricula.
**
Ridley, Michael. (2024). Explainable AI: Implications for Libraries and Archives. Future of Archives and Libraries: How Technology and AI are (Re)Shaping Heritage Institutions. Library and Archives Canada, Ottawa, September 5, 2024.
**
Ridley, Michael (2024, April 25). The explainability imperative. Information Matters, 4(4). https://informationmatters.org/2024/04/the-explainability-imperative/
ABSTRACT
This short piece responds to implications of the question: If artificial intelligence is so smart, why can't it explain itself?
**
Ridley, Michael (2024). Human‐centered explainable artificial intelligence: An Annual Review of Information Science and Technology (ARIST) paper. Journal Of The American Society For Information Science, 1–23. https://doi.org/10.1002/asi.24889
ABSTRACT
Explainability is central to trust and accountability in AI applications. The field of human-centered explainable AI (HCXAI) arose as a response to mainstream explainable AI (XAI) which was focused on algorithmic perspectives and technical challenges, and less on the needs and contexts of the non expert, lay user. HCXAI is characterized by putting humans at the center of AI explainability. Taking a sociotechnical perspective, HCXAI prioritizes user and situational contexts, preferences reflection over acquiescence, and promotes the actionability of explanations. This review identifies the foundational ideas of HCXAI, how those concepts are operationalized in system design, how legislation and regulations might normalize its objectives, and the challenges that HCXAI must address as it matures as a field.
**
Ridley, Michael (2024). Prototyping expert systems in reference services (1980–2000): experimentation, success, disillusionment, and legacy. Library & Information History 40.1 (2024): 46–67 https://doi.org/10.3366/lih.2024.0165
ABSTRACT
In the late twentieth century librarians prototyped expert systems in reference services in order to respond to the reference ‘crisis’ of the time and to harness the power of emerging artificial intelligence (AI) technologies. Creating intelligent systems required librarian designers to codify the expertise of reference librarians and the resources of reference services into knowledge representation mechanisms suitable for inferences by the system. In this process, librarians explored the theoretical and pragmatic bases of reference and experimented with how to implement them in AI. The successes and failures of these prototypes reveal how librarians felt about these new technologies and how they might transform libraries. While expert systems in libraries failed and were abandoned by the end of the century, lessons and insights from this seminal work can inform current activities in the application of machine learning.
We use cookies to analyze website traffic and optimize your website experience. By accepting our use of cookies, your data will be aggregated with all other user data.